Case Study:
Improving Direct Mail Campaigns
The Challenge
Our client commissioned 25 affinity models to assist in improving the results of their direct mail campaigns. The models were to be based on survey data with approximately 35,000 observations. The organization is quite large, and executes marketing campaigns to approximately 5,000,000 individuals (about 20% of the database) with a frequency of 24 times per year. Random mailings across the database performed at a 1.25% response rate with an average value of about $13.50 per responder, and a fully burdened mailing cost of $0.40 per piece. With this volume and overall cost of the direct mail program, there is quite a bit of room for improving efficiency through analytics.
“LityxIQ provides an extremely efficient and cost-effective platform for building and deploying dozens of models.”
– Paul Maiste, President & CEO, Lityx
The Solution
The Results
- 90% savings in development costs for series of 25 predictive models
- Improved model lift using PredictIQ in less than 10% of the time
- Projected incremental 76,000 responses and $1.72 million customer value
Get Started
with Lityx
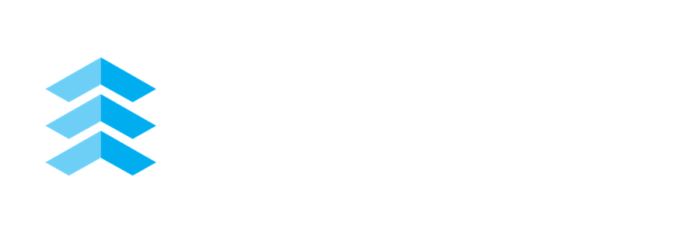