Case Study:
Predict Customer Behavior
International Grocer
The Client
Our client was a retail industry consulting firm delivering analytics and modeling to predict customer behavior. Collaboratively, we engaged with an international online grocery business which was currently successful but facing stiff competition and new challenges.
“We are extremely thankful to Lityx for the valuable guidance and results provided to our client.”
– Project Manager, International Consultancy
The Challenge
The grocery firm had compiled a large detailed data warehouse with information such as customer demographics, line item transactions, marketing activity and responses, customer survey data, and more. The data was not being leveraged beyond simple business intelligence.
Recently, it was noticed that a growing number of customers were making a single visit and not returning. Loyalty was decreasing and churn was increasing. The goal was to increase sales and profitability through increased customer visits (loyalty) and increased spend per visit. In addition, it was increasingly important to identify at-risk customers early in their lifecycle so that action could be taken to make them more loyal.
“It makes something that’s very difficult accessible, and I think that is really a good thing for our clients. I’m really happy that we can bring it to them at a price which is right.”
The Solution
The Results
- Churn models with 330% lift in identifying at-risk customers
- 265% lift in predicting re-purchase behavior following first visit
- Easy capability to build modeling dataset for any historic point-in-time
Get Started
with Lityx
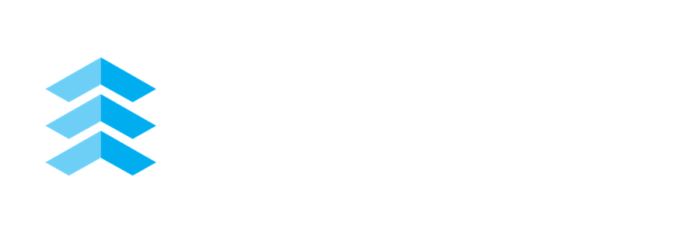